Category: nltk
NLTK stop words
Python and NLTK sent_tokenize
nltk stemming
nltk tags
The module NLTK can automatically tag speech.
Given a sentence or paragraph, it can label words such as verbs, nouns and so on.
NLTK - speech tagging example
The example below automatically tags words with a corresponding class.
|
Related course
Easy Natural Language Processing (NLP) in Python
This will output a tuple for each word:
where the second element of the tuple is the class.
The meanings of these speech codes are shown in the table below:
We can filter this data based on the type of word:
|
which outputs:
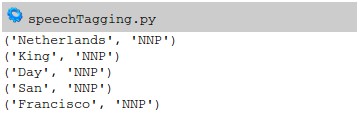
The classes include past tense, present. Using this technique we can quickly derive meaning from a text.